Abstract
Amid mass spread of climate misinformation and disinformation on social media platforms, climate journalists attempt to disseminate accurate climate change information via social media. But when social media algorithms uplift shocking and sometimes untrue content, it can be difficult for climate journalists to engage audiences online. This study uses a mixed-methods approach to discern which characteristics influence engagement levels in tweets from climate journalists. Through computational sentiment analysis, topic modeling, and qualitative coding of tweets, as well as interviews with journalists and experts, this study attempts to identify key factors in user engagement with climate journalism tweets. The study finds that neither positive nor negative sentiment are strongly correlated with engagement metrics. This finding confirms the beliefs of journalists interviewed in the present study and contradicts the views of some experts.
Research Questions
RQ1: Do certain sentiments correlate with higher engagement levels?
RQ2: Do certain topics arise in tweets from climate journalists?
RQ3: Do process-related conceptual patterns exist among higher-engagement tweets?
RQ4(a): Do climate journalists take note of engagement levels on Twitter?
RQ4(b):What kind of engagement on Twitter is substantive for climate journalists?
RQ5: What kind of tweets do climate journalists perceive to perform well?
RQ6: How would climate journalists advise others in the field to create an engaging Twitter presence?
Data Collection
From a sample of 50 climate journalists across the country, 15 journalists were selected at random. Those 15 climate journalists’ entire history of tweets was collected using the Twitter API. They were also contacted for interview, along with another sample of 15 random journalists.
For sentiment analysis and topic modeling, the data was pre-processed to make it machine-readable. This included standard NLP pre-processing with the Python NLTK library. Invalid data points and tweets with fewer than 100 characters were removed from the dataset and punctuation, mentions and URLs were removed from individual tweets.
To extract high-engagement events for process coding, Twitter’s public engagement metrics, retweet, like, reply and quote, were categorized into “popularity-centric” engagement and “commitment-centric” engagement, following this study from New Media & Society. Retweet count and like count were averaged to find a popularity-based engagement score and reply count and quote count were averaged to find a commitment-based engagement score. The top five high-engagement tweets for each metric were collected fo each journalist. This process resulted in two datasets of 75 tweets used for process coding.
Mixed-Methods Approach
This study aims to answer questions about how climate journalists can engage with audiences on Twitter, through interviews with journalists and experts, data analysis of tweets from climate journalists and process coding of those tweets.
Interviews
Interviews were first conducted with eight climate journalists from across the country and two experts from centers for climate change communication. These interviews informed later research, with two main takeaways:
- Experts suggested solutions-based journalism would perform well on Twitter, whereas journalists expected no difference in engagement levels between solutions-oriented tweets and tweets with a more negative outlook on climate change
- Experts and journalists agreed that downscaling climate change to smaller and more relatable examples, such as its impacts on specific regions or specific crops, would engage audiences
Solutions-based journalism and downscaling climate change are both tactics that have been proven effective in traditional climate journalism output by news outlets. However, journalists had expressed that the Twitter algorithm may have more influence than journalistic practices on which tweets perform well.
Sentiment Analysis
A sentiment analysis of the tweets from 15 climate journalists was performed, to test experts’ theory that solutions-based journalism would have high engagement levels on Twitter. After cleaning the dataset and removing outliers, the correlation coefficients below were calculated from a sample of 36,982 tweets. The sentiment analysis was done using Python’s TextBlob library, which assigns each tweet a polarity score and a subjectivity score. Correlation coefficients were calculated between these sentiment scores and Twitter’s public metrics, retweet count, like count, reply count and quote count. The table below shows those results.
Subjectivity score | Polarity score | |
Retweet count | -0.009775 | -0.029019 |
Like count | 0.035766 | 0.016064 |
Reply count | 0.035766 | 0.016064 |
Quote count | -0.007015 | -0.003197 |
Polarity score describes the traditional sentiment of a text sample, i.e. how positive or negative its language is. The following charts depict some of the correlations between polarity score and public metrics.
The analysis found that sentiment bears little weight on a tweet’s engagement levels, which aligns with interviewed journalists’ expectations. This finding contradicts what experts had expected and traditional journalistic practices for climate reporting.
Topic Modeling
Topic modeling was also performed on the tweets from 15 climate journalists, with an LDA Model from Python’s Gensim library. The model extracted 20 topics with five keywords each after passing over a dataset of 84,102 tweets 50 times.
Because tweets are limited to 280 characters, there are few words in each text sample from the start. After removing stop words, it is likely the model had few word combinations to consider. For this reason, 10 out of the 20 generated topics were not viable. The remaining 10 topics are presented in the table below.
Topic | Keywords | Explanation (This topic seems to be about…) |
Firsts | time, new, many, first, wonder | … news events when something happens for the first time. |
Articles | this, was, story, think, good | … promoting news stories. |
Pleasantries | thank, great, well, tweet, friend | … kind words exchanged between friends. |
Sea level rise | come, rise, bay, level, mind | … sea level rise, especially in a bay. |
Praise | love, lol, this, look, like | … admiring some entity. |
California drought | Fresno, California, run, drought, health | … drought in Fresno, California. |
Climate change | climate, say, and, change, this | … climate change news. |
Daily plans | big, final, went, park, tomorrow | … day-to-day plans of a tweet’s author. |
Political campaigns | lead, agree, track, represent, lose | … news about political campaigns. |
Colorado river | Colorado, river, water, oh, video | … the Colorado river. |
The results show that climate journalists often tweet about climate news or other news events and use Twitter to interact with other users.
Process Coding
Once interviews and data analysis had not yielded direct indications of which kinds of tweets from climate journalists performed well, researchers turned to manual inspection to observe patterns in high-engagement tweets. The two datasets of high-engagement tweets were manually reviewed by researchers to extracts actions taken by journalists in those tweets. Those actions, or processes, can be seen below.
Process-related concepts found in tweets with high popularity-centric engagement metrics
Process code | Count | Explanation (Tweets that…) |
Self-promoting | 28 | … linked to an article by the tweet’s author. |
Reporting | 24 | … spoke of a news event. |
Quoting | 12 | … quoted a person. |
Imaging | 12 | … attached photographs. |
Celebrating | 11 | … spoke about a job change or a job-related award. |
Shocking | 10 | … spoke dramatically of a concept or event presently occurring. |
Quantifying | 8 | … included quantities or statistics. |
Frightening | 6 | … spoke dramatically of a concept or event predicted to occur in the future. |
Questioning | 4 | … questioned a phenomenon or the words or actions of a person or institution. |
Clipping | 4 | … attached screenshots from articles. |
Broadcasting | 4 | … attached videos. |
Mocking | 4 | … spoke satirically of a person, creation, phenomenon or institution. |
Recounting | 4 | … relayed an anecdote or personal experience. |
Visualizing | 3 | … attached charts, graphics or maps. |
Downscaling | 3 | … related an overarching concept to a more specific experience. |
Criticizing | 3 | … spoke negatively of a person, creation, phenomenon or institution. |
Praising | 3 | … spoke positively of a person, creation, phenomenon or institution. |
Process-related content found in tweets with high commitment-centric engagement
Process code | Count | Explanation (Tweets that…) |
Self-promoting | 25 | … linked to an article by the tweet’s author. |
Celebrating | 14 | … spoke about a job change or a job-related award. |
Imaging | 13 | … attached photographs. |
Reporting | 12 | … spoke of a news event. |
Shocking | 8 | … spoke dramatically of a concept or event occurring at the time the tweet was made. |
Quantifying | 8 | … included quantities or statistics. |
Recounting | 8 | … that relayed an anecdote or personal experience. |
Quoting | 7 | … quoted a person. |
Frightening | 6 | … spoke dramatically of a concept or event predicted to occur in the future. |
Mocking | 4 | … spoke satirically of a person, creation, phenomenon or institution. |
Visualizing | 4 | … attached charts, graphics or maps. |
Asking | 4 | … sought responses to a question. |
Sourcing | 4 | … sought individuals to speak about an event or experience. |
Clipping | 3 | … attached screenshots from articles. |
The charts below show the distributions of various processes within each high-engagement dataset.
The results reveal that high-engagement tweets tend to show journalists promoting their work, reporting and celebrating career milestones. There were several instances of high-engagement tweets with frightening or shocking information about climate change, but there were no tweets in either dataset that focused on solutions to climate change. There were three instances of downscaling climate change in the popularity-centric dataset, but it was not a prevailing theme in high-engagement tweets.
Discussion
This study suggests that the traditional rules of effective climate journalism may not apply to social media platforms. However, this was one small-scale study. In the future, conducting a similar study on a larger scale could yield more interesting results. Furthermore, journalists and experts agree that they use Twitter less since its purchase by Elon Musk and that they may stop use of the app altogether in the near future. Future studies may wait and see which platform replaces Twitter as journalists’ social media of choice. Mastodon is one platform that is gaining popularity among journalists, and may be suitable for future studies about effective social media practices by climate journalists.
By
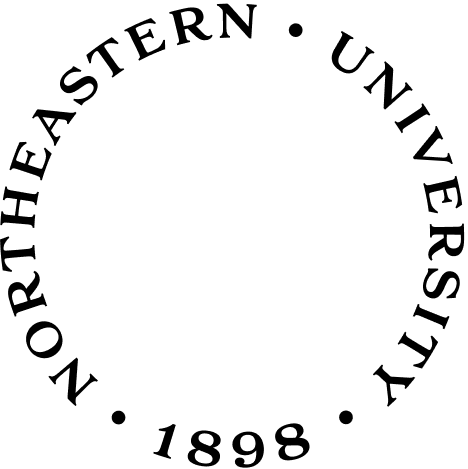
Annetta Stogniew